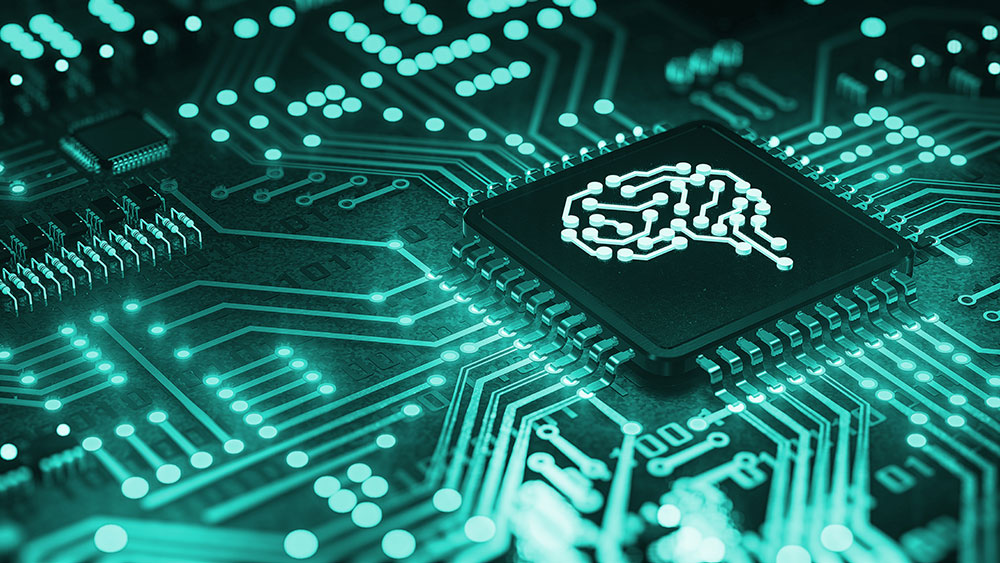
Dr. Jiang Hu, professor in the Department of Electrical and Computer Engineering at Texas A&M University, is working to revitalize electronic design automation (EDA) technology with machine-learning techniques to keep pace with the ever-growing chip design complexity.
EDA is a category of software tools used to design electronic systems such as integrated circuits. Nowadays, chip complexity is extremely high and is continuing to advance at a very fast rate. Current techniques used for EDA are limited in that they don't offer the capability of knowledge reuse. Another limitation is that chip design has many complicated steps, and conventional electronic design automation techniques are centered on point solutions that aim to address a single task at a time. Alternatively, machine learning incorporates past designs and allows for updates and modifications based on those known experiences rather than starting from point-A each time. Much like the human brain, machine learning can build upon experiences and integrate what is learned or gathered to make progress.
“Machine learning is quite different from the conventional techniques in the sense that the conventional techniques do everything from scratch, while machine learning has the capability to extract knowledge from prior designs and reuse the knowledge, which is much more efficient,” Hu said.
Hu is leading a collaborative team of four faculty members from two universities with individual expertise and experience that equips them to address the changing landscape and needs of EDA technology. This project, which is funded by a $1.2 million National Science Foundation grant, aims to customize existing machine-learning techniques for the most efficient use in chip designs to help design faster and more power-efficient chips with a quicker turnaround than is currently possible.
Machine learning can cover the areas that conventional electronic design automation is simply not able to handle.
Collaborators include Dr. Yiran Chen, professor from Duke University, Michael Quinn, associate professor of practice, and Dr. Aakash Tyagi, professor of practice, both from the Department of Computer Science and Engineering at Texas A&M. Chen is an expert in machine learning while Quinn and Tyagi have a collective five decades of industry experience in chip design and verification.
Hu began studying the integration of machine learning into EDA four years ago and was drawn to the field due to its impact across many disciplines and industries.
“If you look at the progress of chip design technology and electronic design automation in the past several decades, there have been mostly small steps, you know, but this is something big; it is fundamental,” Hu said. “Machine learning can cover the areas that conventional electronic design automation is simply not able to handle.”
As part of this project, Hu and his team are also bringing in students, including women and under-represented minorities, with interdisciplinary skills to learn and gain valuable experience in this area of study.