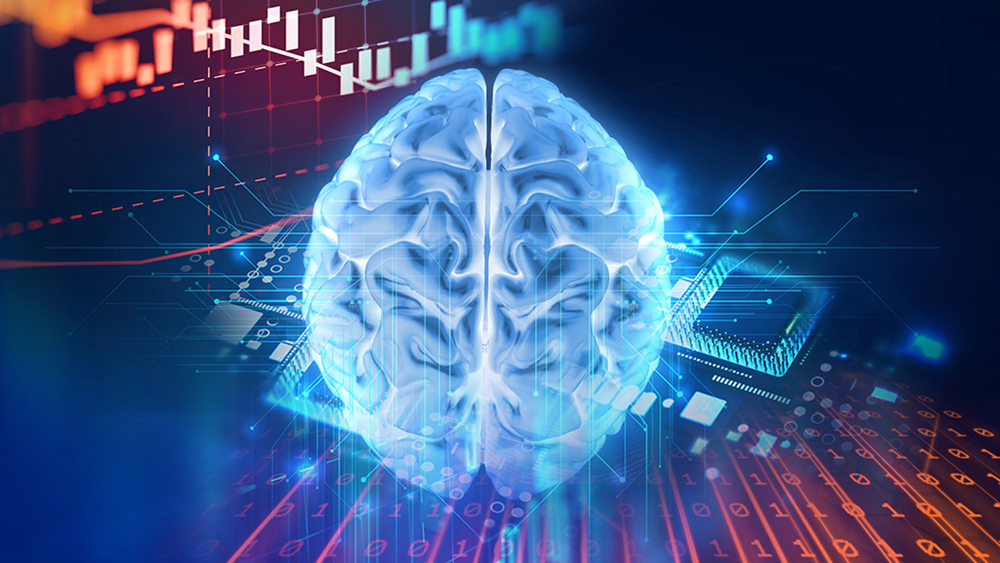
Ever wish your computer could think like you do or perhaps even understand you?
That future may not be now, but it’s one step closer, thanks to a Texas A&M University-led team of scientists and engineers and their recent discovery of a materials-based mimic for the neural signals responsible for transmitting information within the human brain.
The multidisciplinary team, led by Dr. Sarbajit Banerjee, professor in the chemistry department, in collaboration with Dr. R. Stanley Williams, professor in the electrical and computer engineering department, and additional colleagues across North America and abroad, has discovered a neuron-like electrical switching mechanism in the solid-state material β’-CuxV2O5 — specifically, how it reversibly morphs between conducting and insulating behavior on command.
The team was able to clarify the underlying mechanism driving this behavior by taking a new look at β’-CuxV2O5, a remarkable chameleon-like material that changes with temperature or an applied electrical stimulus. In the process, they zeroed in on how copper ions move around inside the material and how this subtle dance in turn sloshes electrons around to transform it. Their research revealed that the movement of copper ions is the linchpin of an electrical conductivity change that can be leveraged to create electrical spikes in the same way that neurons function in the cerebral nervous system — a major step toward developing circuitry that functions like the human brain.
Their resulting paper, “Metal-Insulator Transitions in β’-CuxV2O5 Mediated by Polaron Oscillation and Cation Shuttling,” features Texas A&M chemistry graduate students Abhishek Parija (now at Intel Corporation), Justin Andrews and Joseph Handy as first authors. It is published in the Cell Press journal Matter.
The nuances of neuromorphic computing
In their quest to develop new modes of energy efficient computing, the broad-based group of collaborators is capitalizing on materials with tunable electronic instabilities to achieve what’s known as neuromorphic computing, or computing designed to replicate the brain’s unique capabilities and unmatched efficiencies.
“Nature has given us materials with the appropriate types of behavior to mimic the information processing that occurs in a brain, but the ones characterized to date have had various limitations,” Williams said. “The importance of this work is to show that chemists can rationally design and create electrically active materials with significantly improved neuromorphic properties. As we understand more, our materials will improve significantly, thus providing a new path to the continual technological advancement of our computing abilities.”
While smart phones and laptops seemingly get sleeker and faster with each iteration, Parija notes that new materials and computing paradigms freed from conventional restrictions are required to meet continuing speed and energy-efficiency demands that are straining the capabilities of silicon computer chips, which are reaching their fundamental limits in terms of energy efficiency. Neuromorphic computing is one such approach, and manipulation of switching behavior in new materials is one way to achieve it.
“The central premise — and by extension the central promise — of neuromorphic computing is that we still have not found a way to perform computations in a way that is as efficient as the way that neurons and synapses function in the human brain,” said Andrews, a NASA space technology research fellow. “Most materials are insulating (not conductive), metallic (conductive) or somewhere in the middle. Some materials, however, can transform between the two states: insulating (off) and conductive (on) almost on command.”
By using an extensive combination of computational and experimental techniques, Handy said the team was able to demonstrate not only that this material undergoes a transition driven by changes in temperature, voltage and electric field strength that can be used to create neuron-like circuitry, but also comprehensively explain how this transition happens. Unlike other materials that have a metal-insulator transition, this material relies on the movement of copper ions within a rigid lattice of vanadium and oxygen.
“We essentially show that a very small movement of copper ions within the structure brings about a massive change in conductance in the whole material,” Handy added. “Because of this movement of copper ions, the material transforms from insulating to conducting in response to external changes in temperature, applied voltage or applied current. In other words, applying a small electrical pulse allows us to transform the material and save information inside it as it works in a circuit, much like how neurons function in the brain.”
Andrews likens the relationship between the copper-ion movement and electrons on the vanadium structure to a dance.
“When the copper ions move, electrons on the vanadium lattice move in concert, mirroring the movement of the copper ions,” Andrews said. “In this way, incredibly small movements of the copper ions induce large electronic changes in the vanadium lattice without any observable changes in vanadium-vanadium bonding. It’s like the vanadium atoms ‘see’ what the copper is doing and respond.”
Demands, deficiencies and the global data glut
Transmitting, storing and processing data currently accounts for about 10% of global energy use, but Banerjee said extrapolations indicate the demand for computation will be many times higher than the projected global energy supply can deliver by 2040. Exponential increases in computing capabilities therefore are required for transformative visions, including the “internet of things,” autonomous transportation, disaster-resilient infrastructure, personalized medicine and other societal grand challenges that otherwise will be throttled by the inability of current computing technologies to handle the magnitude and complexity of human- and machine-generated data. He said one way to break out of the limitations of conventional computing technology is to take a cue from nature — specifically, the neural circuitry of the human brain, which vastly surpasses conventional computer architectures in terms of energy efficiency and also offers new approaches for machine learning and advanced neural networks.
“To emulate the essential elements of neuronal function in artificial circuitry, we need solid-state materials that exhibit electronic instabilities, which, like neurons, can store information in their internal state and in the timing of electronic events,” Banerjee said. “Our new work explores the fundamental mechanisms and electronic behavior of a material that exhibits such instabilities. By thoroughly characterizing this material, we have also provided information that will instruct the future design of neuromorphic materials, which may offer a way to change the nature of machine computation from simple arithmetic to brain-like intelligence while dramatically increasing both the throughput and energy efficiency of processors.”
Because the various components that handle logic operations, store memory and transfer data are all separate from each other in conventional computer architecture, Banerjee said they are plagued by inherent inefficiencies regarding both the time it takes for information to be processed and how physically close together device elements can be before thermal waste and electrons “accidentally” tunneling between components become major problems. By contrast, in the human brain, logic, memory storage and data transfer are simultaneously integrated into the timed firing of neurons that are densely interconnected in 3D fanned-out networks. As a result, the brain’s neurons process information at 10 times lower voltage and an almost 5,000 times lower synaptic operation energy in comparison to silicon computing architectures. To come close to achieving this kind of energetic and computational efficiency, he said new materials are needed that can undergo rapid internal electronic switching in circuits in a way that mimics how neurons fire in timed sequences.
Parameters of perfection in principle
Handy notes that the team still needs to optimize many parameters, such as transition temperature and switching speed along with the magnitude of the change in electrical resistance. By determining the underlying principles of the metal-insulator transition in β’-CuxV2O5 as a prototype material within an expansive field of candidates, however, the team has identified certain design motifs and tunable chemical parameters that ultimately prove useful in the design of future neuromorphic computing materials, a major endeavor that has been seeded by the Texas A&M X-Grant program.
“This discovery is very exciting because it provides fertile ground for the development of new design principles for tuning materials properties and also suggests exciting new approaches to researchers in the field for thinking about energy efficient electronic instabilities,” Parija said. “Devices that incorporate neuromorphic computing promise improved energy efficiency that silicon-based computing has yet to deliver, as well as performance improvements in computing challenges like pattern recognition — tasks that the human brain is especially well-equipped to tackle. The materials and mechanisms we describe in this work bring us one step closer to realizing neuromorphic computing and in turn actualizing all of the societal benefits and overall promise that comes with it.”
The multi-year project incorporates team members from four disciplines (chemistry, physics, materials science and engineering, and electrical and computer engineering) and researchers from Texas A&M, Lawrence Berkeley National Laboratory, the University at Buffalo, Binghamton University and Texas A&M University at Qatar, while also relying on work performed at Berkeley Lab’s The Molecular Foundry and the Advanced Light Source, the Advanced Photon Source at Argonne National Laboratory and the Canadian Light Source. The research was funded primarily by the National Science Foundation, with additional support from a Texas A&M X-Grant and the Qatar National Research Fund.
This article originally appeared on the College of Science's news site.