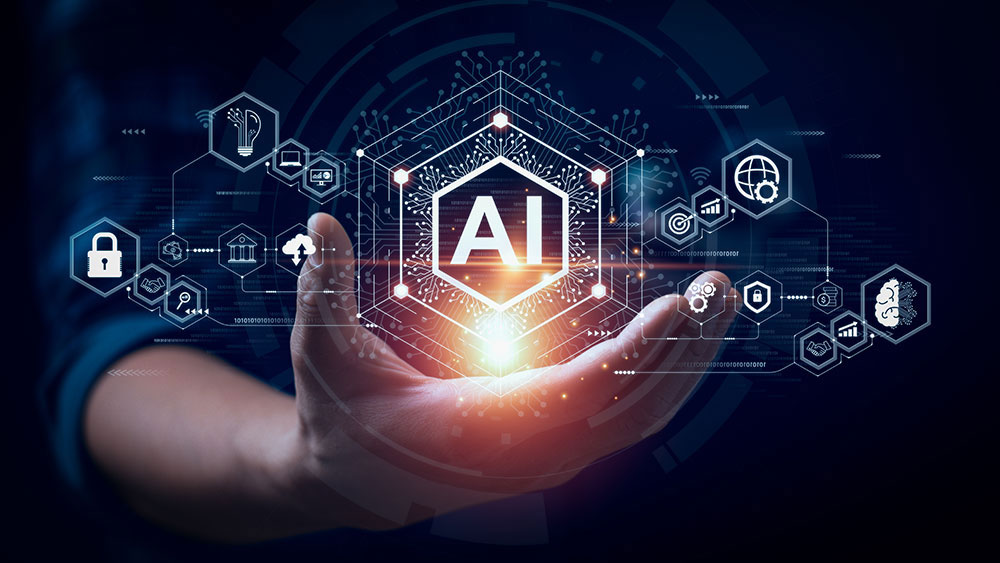
What if artificial intelligence could be used to spur discovery in areas such as biotechnology, drug discovery and fluid dynamics? Using geometric graphs and innovative methodologies, AI can solve fundamental problems in basic natural science. The possibilities are endless in this relatively new field known as AI for science.
In the Department of Computer Science and Engineering at Texas A&M University, Dr. Shuiwang Ji recently received a National Science Foundation grant to research 3D graphs and artificial intelligence.
The professor aims to develop a methodology to represent molecules and proteins using 3D or geometric graphs to predict their behavior and properties. Once created, this methodology could help solve problems in physics, fluid dynamics and biotechnology.
“We try to use AI to understand the physical world we live in,” Ji said. “The findings of this research may take time to become practical, but once that happens and we develop a powerful AI method, it can be applied to many different areas because this is fundamental research. It’s similar to when the discovery of the electron led to electricity.”
Completeness, efficiency and applications
The challenge lies in the uniqueness of Ji’s project: bridging completeness, efficiency and applications in 3D graphs. The application aspect refers to applying Ji’s methodology to molecular dynamics, molecular simulations and drug discovery. Completeness refers to capturing complete information, and efficiency refers to processing large inputs. With this kind of work, there are usually trade-offs.
“Traditionally, if you want to capture complete input information, the process will be quite slow to train this network,” Ji said. “On the other hand, if you want the training to be fast, you cannot capture complete information. I aim to develop a method that will be both and solve different applications in molecules, proteins and material science. This might be the first time these three things are being put together.”
We use AI to solve problems in computer science, chemistry, fluid dynamics and material science, but we are not experts in these fields. It would be good to collaborate with these individuals to see what challenges they face that we can try to solve.
Understanding graphs and real-world applications
Graphs are used to represent certainties in the physical world. For instance, a standard graph could represent a social network, where each node is a person, and the edges represent relationships; if Person A is in an email communication with Person B, there is an edge from A to B.
“In science, we can characterize a problem as a geometric graph,” Ji said. “This is very different from standard graphs because each node will also have a location or coordinate in 3D space. You can consider a molecule as a geometric graph, where each atom is a node and chemical bonds characterize their relationships. Each atom also has a location in 3D space, which gives you the 3D geometry of these molecules. How the molecule will perform critically depends on 3D geometry.”
A material that geometric graphs can represent is drugs, which are made of molecules. A drug will have a 3D shape, dock to a receptor and bind a protein like a key to a lock. The 3D geometry of the drug is important because it can be formulated as a prediction problem to find out if the drug can be used as an antibiotic or not.
“The drug industry is interested in molecular dynamics, and a similar model has been used to screen a large potential antibiotic database,” Ji said. “They set out to identify a molecule that could potentially be used as an antibiotic, validated their findings in the lab and actually identified a new antibiotic.”
The applications of this project include not only drug discovery but also material science, partial differential equations and aerospace engineering, to name a few. Ji plans to focus on the methodology rather than one area of study, inviting collaboration from experts in a wide range of fields to lend insight into this work and solve many problems.
“This is a highly interdisciplinary area,” Ji said. “We use AI to solve problems in computer science, chemistry, fluid dynamics and material science, but we are not experts in these fields. It would be good to collaborate with these individuals to see what challenges they face that we can try to solve.”
Where it all began
Though Ji begins this research project on the first of August, he has been working on AI for science for a few years. He even led a technical survey review paper laying the foundation for this research project, “Artificial Intelligence for Science in Quantum, Atomistic, and Continuum Systems.” Similar to the project's goal, this 263-page paper invited collaboration from researchers of various fields, including contributions from 63 authors and 14 universities.
“Since AI for science is a relatively new area, there is not a lot of literature on the subject to reference,” Ji said. “The project is a subset of the paper and focuses on molecular dynamics/representations, representing molecules and proteins in terms of geometric graphs. The paper lays a foundation for this project to move forward.”