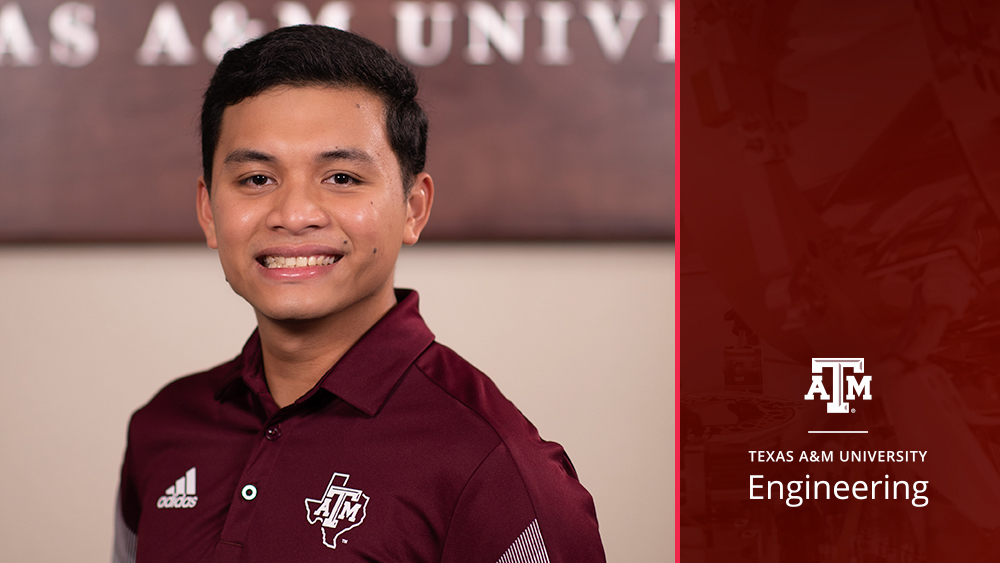
Eliminating by design the possibility of reactivity-initiated accidents in nuclear reactors led to the development of subcritical systems with inherent safety characteristics. In these systems, energy generation can be regulated and controlled by an external source.
Due to this advantage, a subcritical reactor driven by a powerful neutron source, such as an accelerator-driven system (ADS), has been proposed as an alternative technology to commercial nuclear energy generation or as a dedicated system for transmuting nuclear waste to reduce its long-term radiotoxicity.
However, there are currently no operating ADSs capable of industrial-scale waste transmutation, creating a need for experimentation and testing that can be costly and time-consuming.
As part of the Foreign Graduate Scholarship Program in the Department of Science and Technology-Science Education Institute of the Republic of the Philippines, Ronald Gatchalian, a graduate student in the Department of Nuclear Engineering at Texas A&M University, is using machine-learning techniques to predict the physics parameters of a source-driven reactor configuration in a subcritical domain, reducing experimentation costs and time.
This research was published in Annals of Nuclear Energy.
To conduct his research, Gatchalian is focused on modeling the newly reopened Philippine Research Reactor-1 Subcritical Assembly for Training, Education, and Research Reactor — the country's sole nuclear reactor training facility.
Reactors normally operate at a critical state, meaning the system is in a steady state, and the fission chain reaction is self-sustained. When reactors are operated at a subcritical state, the system cannot sustain the fission reactions on its own — it must be supplied with neutrons, meaning it relies on its external source of neutrons for power. This is why it is referred to as a source-driven reactor.
This dependence on the source makes the reactor easier to control and operate safely because the chain reaction dies once the source is removed. This presents a significant advantage over critical reactors in terms of inherent safety because the possibility of a criticality accident is eliminated by design.
Different sources can be chosen for subcritical systems that fall on different parts of the neutron spectrum — some are lower energy, and some are higher energy. For example, Californium-252 is lower energy than Deuterium-Deuterium, Deuterium-Tritium and other accelerator-based sources.
Additionally, the positioning of the source within the reactor can alter the flux level in the core on which the system performance is based. However, determining the optimal source and position requires extensive and costly experimental testing.
To combat this issue, Gatchalian has developed a machine-learning model to gain insight into the physics parameters and behaviors of nuclear reactors.
“Using my technique, I can simulate experiments in advance, so when the experiment is completed, it can be checked against this machine-learning model to see if this is within expectation,” said Gatchalian.
In his research, Gatchalian found that in a well-moderated or thermal system, it's better to have a neutron source on the lower end of the energy spectrum. This is because neutron leakage is reduced, improving neutron source efficiency and leading to higher steady-state flux. This behavior differs from a system with two-zone spectral and spatial domains.f
“In a critical system, the external neutron source is only needed to kickstart the reaction and is not the main driver because the system is self-sustaining,” said Gatchalian. “But in a subcritical system, it has a big effect because the reactor performance depends on the neutron source.”
A subcritical system functions below criticality. Suppose a system is intended for subcritical operation. In that case, it shouldn’t reach criticality because it lacks the appropriate safety systems as justified by graded-approach — this is referred to as the margin from criticality.
“There's a trade-off to the margin of criticality — the closer to criticality, the more neutrons, and the benefit of those neutrons then translates to shorter counting time, or capability to perform experiments that require higher flux,” he said. “Critical systems adhere to more stringent regulations as commensurate of its potential hazard, and these regulations can differ in each country.”
The substantial safety margin is achieved by limiting parameters such as fissile material loading, moderation, absorption and reflection, where typically, fissile material loading is fixed, so there is not enough fuel to achieve criticality.
The ability to predict the physics parameters has several benefits, including improved utilization of the reactor and efficient design of experiments while simultaneously moving the field further in optimization.
“For example, if you need to determine performance and safety metrics from reaction rates or detector counts, machine learning can predict those important parameters that are not directly observable,” he said. “This technology also makes room for optimization because running high-fidelity codes can be computationally expensive. Machine learning can predict these quantities, which can be used as an alternate tool for research.”
This work was completed alongside Dr. Pavel Tsvetkov, associate professor and director of graduate programs for the nuclear engineering department.
“Due to safety considerations associated with criticality, reliably evaluating subcritical systems is very important,” said Tsvetkov. “This work focuses on this subject by taking advantage of data science methods to resolve analytical challenges. The results will assist in safely operating subcritical assemblies and research reactors before attaining criticality.”
In the future, Gatchalian plans to continue working on his machine-learning framework and develop the technology.
“Building a machine-learning model takes a multitude of computing resources and requires datasets that take time to compile,” he said. “This method can serve as a complementary tool to standard techniques in reactor physics evaluation, and being able to check our results against the program creates more confidence in the experimental results.”