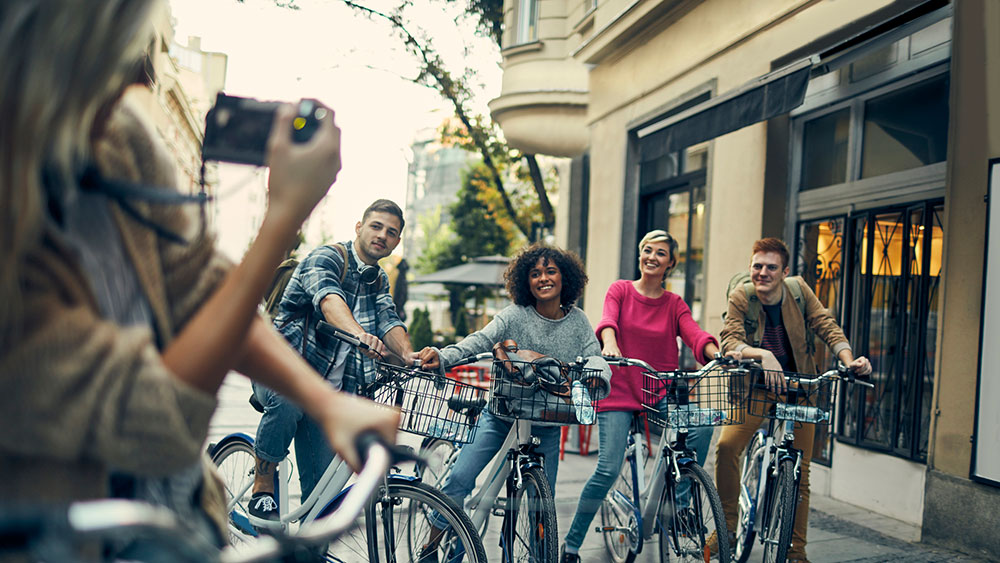
Homophily is the notion that humans tend to preferentially interact and connect with individuals who are like them in some way. In other words, it’s the idea that “birds of a feather flock together.” While traditional research focuses on measuring homophily from the perspective of two-way relationships, such as ones encoded by friendship links in a social network, many human interactions are inherently group interactions and standard tools for measuring homophily do not apply in these settings.
Dr. Nate Veldt, assistant professor in the Department of Computer Science and Engineering at Texas A&M University, along with his collaborators from Cornell University, Dr. Austin R. Benson and Dr. Jon Kleinberg, have developed a mathematical framework using hypergraphs to measure and define homophily in social group interactions.
“While research on homophily has already been very influential in helping us understand connections and interactions from the perspective of two-way interactions and relationships, society is full of multiway interactions and a lot of early research in sociology was focused on understanding how homophily affects group formation and group interactions,” said Veldt. “Providing clearer mathematical measures and computational tools for quantifying homophily in group settings allows us to better address this original motivation for studying homophily.”
The team’s findings have been published in the journal Science Advances.
Rooted in the social sciences, homophily is studied because it serves as a fundamental principle that governs how people interact, behave and connect with one another. It also partially explains how we form friendships and how and why we connect with one another. Those connections are based on various factors such as age, race, gender, education level, religion, aspirations or attitudes.
“If you think of friendships, homophily does not mean I only ever befriend one type of person,” said Veldt. “But if, for example, I disproportionately form friendships with other people of my same age — more than you would expect at random — then you'd say I’m expressing homophily with respect to age in my friendships.”
Previous measures of homophily use a graph model for human interactions. A graph is a mathematical structure that encodes a set of objects (called the nodes) and a set of pairwise relationships between those objects (called edges). For example, edges in a graph can encode the fact that two people are friends in a social network or that one person sends an email to another person.
Graph-based homophily research has been influential in helping researchers understand human connections and interactions. However, looking at society, many of our interactions occur in group settings, such as participating in collaborations at work, conversations on social media or volunteering at events. Graph models do not include valuable information about the size and makeup of the groups people participate in.
There has been a recent surge of interest in modeling different types of complex systems and datasets using hypergraphs, a generalization of graphs that can directly encode multiway relationships. A hypergraph is made up of a set of nodes representing what is being studied and hyperedges, each of which encodes a multiway relationship shared by a group of (possibly more than two) nodes. For example, in a dataset encoding cooking recipes, each ingredient would be a node, and the recipe is the multiway relationship (a hyperedge) that brings them together.
“We have these big, rich modern datasets that are encoding these sorts of multiway relationships, and researchers are finding that a hypergraph can be a very useful way to model a collection of interactions and relationships involving more than two actors at once,” said Veldt.
Using hypergraphs, Veldt and his collaborators developed ways to measure notions of group homophily that cannot be captured by graphs. For example, their framework provides one measure of majority homophily, which is the tendency to participate in group interactions where at least a majority of group participants share a certain attribute (age, gender, political affiliation, etc.). They applied their framework to reveal natural patterns of group homophily based on gender in academic collaborations and group homophily with respect to political affiliation in legislative bill cosponsorships.
Their research also uncovered that there are mathematical properties that are independent of human choices and preferences that must be accounted for in order to properly understand and measure group homophily. For example, some ways to define hypergraph homophily seem intuitive at first and directly generalize existing definitions of graph homophily but are mathematically impossible to satisfy.
“When studying social interactions, it’s important to realize the difference between patterns that you observe because the math requires those patterns to exist and patterns that you see because of underlying sociological phenomena,” said Veldt. “In other words, you need to know about the math in order to draw proper conclusions about the sociology.”
As for future research, one direction the team could go in is measuring the social mechanism of reciprocity, which is the tendency to return benefits.
“For example, if you reply to my emails, I may be more likely to reply to you. Or if someone gives someone a gift, they're more likely to give back in a similar way in the future,” said Veldt. “What does reciprocity look like in group settings? And how do you measure that and study that using new mathematical frameworks?”
In addition to helping researchers understand society better, the principle of homophily has been very useful for designing methods for computational data analysis tasks, such as classifying a set of objects based on their relationships or predicting future interactions. One hope for these new measures of hypergraph homophily is that they will lead to improved methods for these and other tasks in settings where relationships and interactions are inherently multiway.