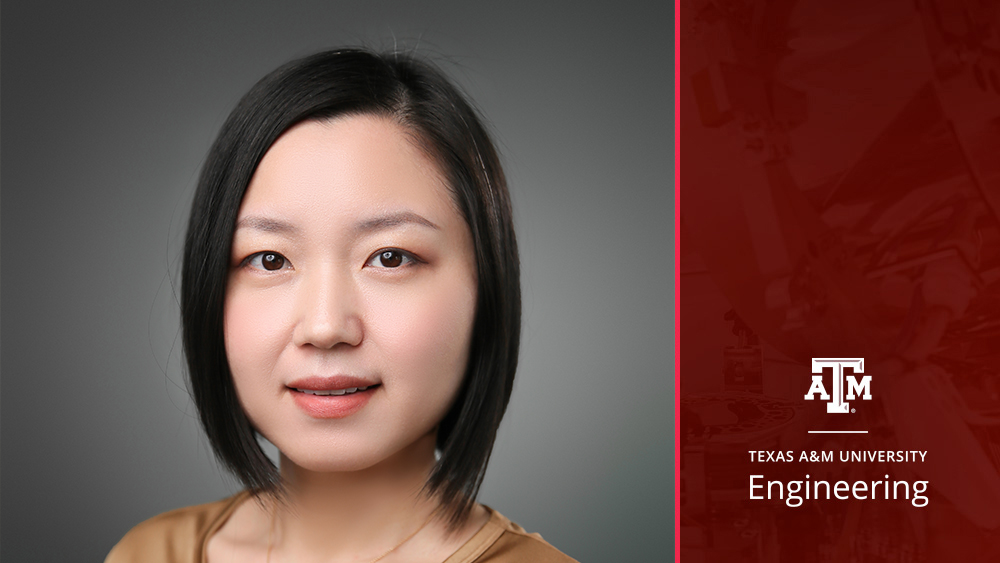
Dr. Na Zou’s research projects have resulted in publications at prestigious venues, and they received the Institute of Electrical and Electronics Engineers Irv Kaufman Award and Texas A&M Institute of Data Science Career Initiation Fellow. |
Image: Courtesy of Dr. Na Zou
Dr. Na Zou, an assistant professor in the Department of Engineering Technology and Industrial Distribution, and Sirui Ding are researchers at Texas A&M University who are working to improve the fairness and effectiveness of machine learning in health care.
Zou's research focuses on developing data-driven models and knowledge discovery to address challenges posed by large-scale, dynamic and networked data in various real-world applications — with some of their research projects resulting in publications on multiple prestigious platforms.
Specifically, Zou is interested in improving fairness in machine learning, interpretable machine learning, transfer learning, and network modeling and inference.
Ding, a third-year doctoral student in the Department of Computer Science and Engineering, specializes in artificial intelligence for health care and is particularly interested in precision health and ethical issues. Ding was recently nominated as one of the Best Student Paper Award finalists at the American Medical Informatics Association (AMIA) 2022 Annual Symposium.
Zou and Ding are currently working on a project that could significantly impact the liver transplant system, which has been criticized for showing discriminatory behavior based on demographics according to research by the National Library of Medicine.
"The current liver transplant system is based on a Model for End-stage Liver Disease (MELD) score to allocate organs," Zou explained. This is a strategy that calculates the likelihood of success based on a patient's lab test results.
But MELD does not consider post-transplant outcomes or organ and donor features.
Ding proposes a fair machine-learning framework to predict graft failure and improve fairness in the liver transplant system.
The framework would ensure that patients with similar medical conditions, but in different demographic groups, have an equal chance of receiving organs.
Their project is sponsored by a National Science Foundation grant to promote equity in the entire health care ecosystem.
They aim to make organ allocation more transparent and non-discriminatory while reducing graft failure rate after transplant treatment.
The project's impact could have the potential to not only improve patient outcomes and promote equitable access to organs, but could also lead to more transparent and non-discriminatory decision-making processes for other organ allocation and medical information systems, such as plasma donation and intensive care unit beds.
"The project, in collaboration with UTHealth Houston and Rice University, is about developing machine-learning algorithms to provide accurate and fair liver assignment to patients," Zou stated.
To provide more context about the importance of their work, Zou and Ding noted that the current disparities in the liver transplant system constitute a significant issue that affects many patients, especially those in disadvantaged demographic groups.
Ding and Zou want to ensure that all patients have an equal opportunity to receive the care they need, regardless of their background or circumstances.
Overall, the researchers’ work is a fundamental step toward creating a more equitable and effective health care system, and their efforts can potentially improve the lives of many patients.
Zou's research focuses on developing data-driven models and knowledge discovery to address challenges posed by large-scale, dynamic and networked data in various real-world applications — with some of their research projects resulting in publications on multiple prestigious platforms.
Specifically, Zou is interested in improving fairness in machine learning, interpretable machine learning, transfer learning, and network modeling and inference.
Ding, a third-year doctoral student in the Department of Computer Science and Engineering, specializes in artificial intelligence for health care and is particularly interested in precision health and ethical issues. Ding was recently nominated as one of the Best Student Paper Award finalists at the American Medical Informatics Association (AMIA) 2022 Annual Symposium.
Zou and Ding are currently working on a project that could significantly impact the liver transplant system, which has been criticized for showing discriminatory behavior based on demographics according to research by the National Library of Medicine.
"The current liver transplant system is based on a Model for End-stage Liver Disease (MELD) score to allocate organs," Zou explained. This is a strategy that calculates the likelihood of success based on a patient's lab test results.
But MELD does not consider post-transplant outcomes or organ and donor features.
Ding proposes a fair machine-learning framework to predict graft failure and improve fairness in the liver transplant system.
The framework would ensure that patients with similar medical conditions, but in different demographic groups, have an equal chance of receiving organs.
Their project is sponsored by a National Science Foundation grant to promote equity in the entire health care ecosystem.
They aim to make organ allocation more transparent and non-discriminatory while reducing graft failure rate after transplant treatment.
The project's impact could have the potential to not only improve patient outcomes and promote equitable access to organs, but could also lead to more transparent and non-discriminatory decision-making processes for other organ allocation and medical information systems, such as plasma donation and intensive care unit beds.
"The project, in collaboration with UTHealth Houston and Rice University, is about developing machine-learning algorithms to provide accurate and fair liver assignment to patients," Zou stated.
To provide more context about the importance of their work, Zou and Ding noted that the current disparities in the liver transplant system constitute a significant issue that affects many patients, especially those in disadvantaged demographic groups.
Ding and Zou want to ensure that all patients have an equal opportunity to receive the care they need, regardless of their background or circumstances.
Overall, the researchers’ work is a fundamental step toward creating a more equitable and effective health care system, and their efforts can potentially improve the lives of many patients.