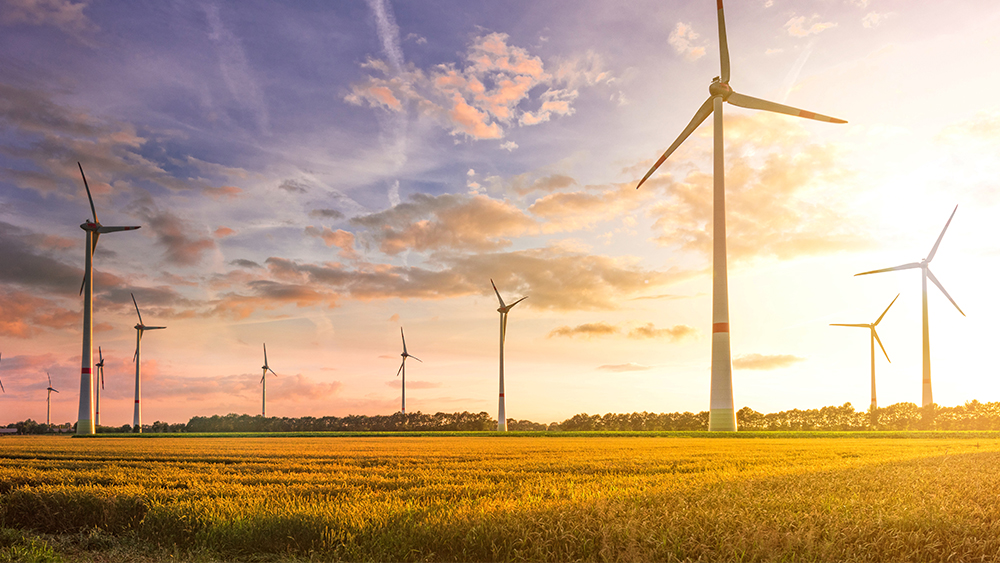
With a global impetus toward utilizing more renewable energy sources, wind presents a promising, increasingly tapped resource. Despite the many technological advancements made in upgrading wind-powered systems, a systematic and reliable way to assess competing technologies has been a challenge.
In a new case study, researchers at Texas A&M University, in collaboration with international energy industry partners, have used advanced data science methods and ideas from the social sciences to compare the performance of different wind turbine designs.
“Currently, there is no method to validate if a newly created technology will increase wind energy production and efficiency by a certain amount,” said Dr. Yu Ding, Mike and Sugar Barnes Professor in the Wm Michael Barnes '64 Department of Industrial and Systems Engineering. “In this study, we provided a practical solution to a problem that has existed in the wind industry for quite some time.”
The results of their study are published in the journal Renewable Energy.
Wind turbines convert the energy transferred from air hitting their blades to electrical energy. As of 2020, about 8.4% of the total electricity produced in the United States comes from wind energy. Further, over the next decade, the Department of Energy plans to increase the footprint of wind energy in the electricity sector to 20% to meet the nation’s ambitious climate goals.
In keeping with this target, there has been a surge of novel technologies, particularly to the blades that rotate in the wind. These upgrades promise an improvement in the performance of wind turbines and, consequently, power production. However, testing whether or how much these quantities will go up is arduous.
One of the many reasons that make performance evaluation difficult is simply because of the sheer size of wind turbines that are often several hundred feet tall. Testing the efficiency of these gigantic machines in a controlled environment, like a laboratory, is not practical. On the other hand, using scaled-down versions of wind turbines that fit into laboratory-housed wind tunnels yield inaccurate values that do not capture the performance of the actual-size wind turbines. Also, the researchers noted that replicating the multitude of air and weather conditions that occur in the open field is hard in the laboratory.
Hence, Ding and his team chose to collect data from inland wind farms for their study by collaborating with an industry that owned wind farms. For their analysis, they included 66 wind turbines on a single farm. These machines were fitted with sensors to continuously track different items, like the power produced by the turbines, wind speeds, wind directions and temperature. In totality, the researchers collected data over four-and-a-half years, during which time the turbines received three technological upgrades.
To measure the change in power production and performance before and after the upgrade, Ding and his team could not use standard pre-post intervention analyses, such as those used in clinical trials. Briefly, in clinical trials, the efficacy of a certain medicine is tested using randomized experiments with test groups that get the medication and controls that did not. The test and the control groups are carefully chosen to be otherwise comparable so that the effect of the medicine is the only distinguishing factor between the groups. However, in their study, the wind turbines could not be neatly divided into the test and control-like groups as needed for randomized experiments.
“The challenge we have here is that even if we choose ‘test’ and ‘control’ turbines similar to what is done in clinical trials, we still cannot guarantee that the input conditions, like the winds that hit the blades during the recording period, were the same for all the turbines,” said Ding. “In other words, we have a set of factors other than the intended upgrades that are also different pre- and post-upgrade.”
Hence, Ding and his team turned to an analytical procedure used by social scientists for natural experiments, called causal inference. Here, despite the confounding factors, the analysis still allows one to infer how much of the observed outcome is caused by the intended action, which in the case of the turbines, was the upgrade.
For their causal inference-inspired analysis, the researchers included turbines only after their input conditions were matched. That is, these machines were subject to similar wind velocities, air densities, or turbulence conditions during the recording period. Next, using an advanced data comparison methodology that Ding jointly developed with Dr. Rui Tuo, assistant professor in the industrial and systems engineering department, the research team reduced the uncertainty in quantifying if there was an improvement in wind turbine performance.
Although the method used in the study requires many months of data collection, Ding said that it provides a robust and accurate way of determining the merit of competing technologies. He said this information will be beneficial to wind operators who need to decide if a particular turbine technology is worthy of investment.
“Wind energy is still subsidized by the federal government, but this will not last forever and we need to improve turbine efficiency and boost their cost-effectiveness,” said Ding. “So, our tool is important because it will help wind operators identify best practices for choosing technologies that do work and weed out those that don't.”
Ding received a Texas A&M Engineering Experiment Station Impact Award in 2018 for innovations in data and quality science impacting the wind energy industry.
Other contributors to the research include Nitesh Kumar, Abhinav Prakash and Adaiyibo Kio from the industrial and systems engineering department and technical staff of the collaborating wind company.
This research is funded by the National Science Foundation and industry.