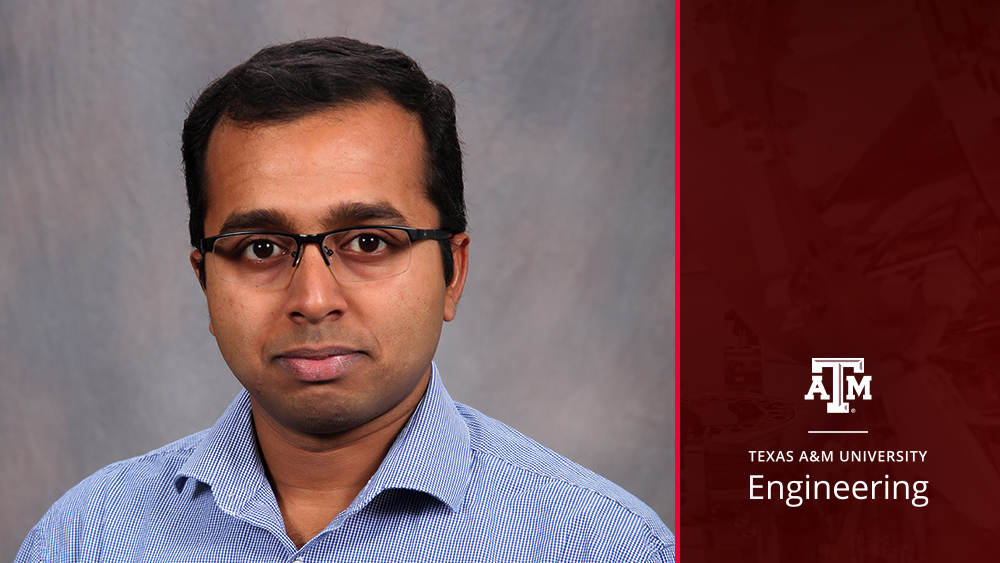
Story Overview
Dr. Dileep Kalathil is working to make the promises of the artificial intelligence evolution attainable by addressing the resiliency, scalability and data efficiency of the system.
Artificial intelligence (AI) and machine learning have transformed our daily lives. Our phones keep getting smarter. We have smart home devices that can control everything in our home by voice command. Even social media platforms and web browsers have a good understanding of what we really want to see and do based on the data provided. In addition to all of this progress, artificial intelligence promises something else – large, societal-scale engineering systems, such as self-driving cars and large-scale power systems, with more capabilities.
Dr. Dileep Kalathil, assistant professor in the Department of Electrical and Computer Engineering at Texas A&M University, is working to make these promises attainable.
Kalathil recently received the Faculty Early Career Development (CAREER) Award from the National Science Foundation (NSF), for his proposal titled "Towards a Principled Framework for Resilient, Data Efficient and Scalable Reinforcement Learning for Control". The NSF CAREER award is one of the most prestigious recognitions given to researchers early in their careers. Kalathil will use his CAREER award to address three major challenges of the AI evolution – resiliency, scalability and data efficiency of the system.
To address these challenges, he is using reinforcement learning principles. Resiliency refers to the robustness and safety integration of an artificial intelligent machine. For example, if a self-driving car is used to driving conditions in Central Texas and there is an unexpected snow day, the adaptability of that vehicle to adjust to those conditions and continue to drive safely is critical.
“This is an area of machine learning that is not very well addressed,” Kalathil said. “And that is what reinforcement learning is about. Reinforcement learning is essentially machine learning for making active decisions.”
Additionally, Kalathil is investigating the issue of scalability so that machine learning systems can be integrated for large-scale technologies such as massive power systems. He is also exploring ways in which progress in this area of machine learning can continue, even with a limited amount of data to go on. In order to make progress, data collection is needed. However, when looking at large-scale systems, data is naturally limited due to cost.
“Suppose you want to design a machine-learning algorithm to control a self-driving car or drone, you will need to fly that drone,” Kalathil said. “However, flying the drone or employing a self-driving car to learn and gather data is not a cheap proposition. You need to deal with the idea that in many cases you will have a really limited amount of data.”
Kalathil will also utilize an experiential learning approach to integrate this reinforcement learning research into his educational curriculum by working with students on the Aggie Deep Racer project, which is a tiny autonomous toy car where reinforcement learning models can be tested. The idea is that the reinforcement learning algorithm can be integrated into the toy car’s system and the application can be put to practice on a real track.
“One thing I believe we should do as an engineering department is to give students the opportunity of experiential learning,” Kalathil said. “They should be able to try things, work on real-world problems and act as engineers.”