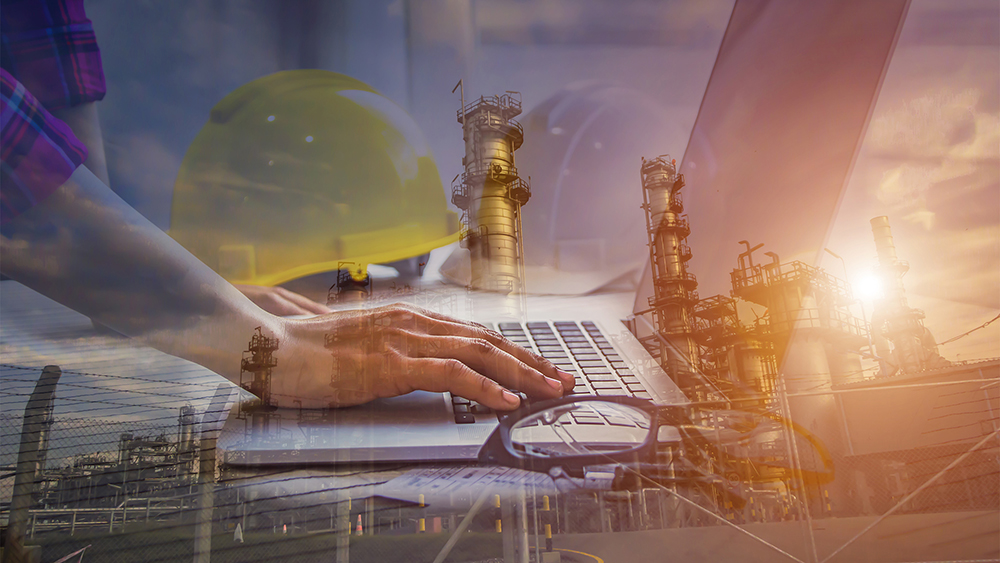
Both a machine-learning algorithm and an engineer can predict if a bridge is going to collapse when they are given data that shows a failure might happen. Engineers can interpret the data based on their knowledge of physics, stresses and other factors, and state why they think the bridge is going to collapse. Machine-learning algorithms generally can't give an explanation of why a system would fail because they are limited in terms of interpretability based on scientific knowledge.
Since machine-learning algorithms are tremendously useful in many engineering areas, such as complex oil and gas processes, Dr. Akhil Datta-Gupta is leading Texas A&M University's participation in a multi-university and national laboratory project started Sept. 2 and initially funded by the U.S. Department of Energy (DOE) with $182,537 to reduce this limitation. He and the other participants will inject science-informed decision-making into machine-learning systems, creating an advanced evaluation system that can assist with the interpretation of reservoir production processes and conditions while they happen.
Hydraulic fracturing operations are complex. Data is continually recorded during production processes so it can be evaluated and modeled to simulate what happens in a reservoir during the injection and recovery processes. However, these simulations are time-consuming to make, meaning they are not available during production and are more of a reference or learning tool for the next operation.
The DOE project will create an advanced system that will quickly sift data produced during hydraulic fracturing operations through physics-enhanced machine-learning algorithms, which will filter the outcomes using past observed experiences, and then render near real-time changes to reservoir conditions during oil recovery operations. These rapid visual evaluations will allow oil and gas operators to see, understand and effectively respond to real-time situations. The time advantage permits maximum production in areas that positively respond to fracturing, and stops unnecessary well drilling in areas that show limited response to fracturing.
"It takes considerable effort to determine what changes occur in the reservoir," said Datta-Gupta, a University Distinguished Professor and Texas A&M Engineering Experiment Station researcher. "This is why speed becomes critical. We are trying to do a near real-time analysis of the data, so engineering operations can make decisions almost on the fly.”
The Texas A&M team’s first step will focus on evaluating shale oil and gas field tests sponsored with DOE funding and identifying the machine-learning systems to use as the platform for the project. Next, they will upgrade these systems to merge multiple types of reservoir data, both actual and synthetic, and evaluate each system on how well it visualizes underground conditions compared to known outcomes.
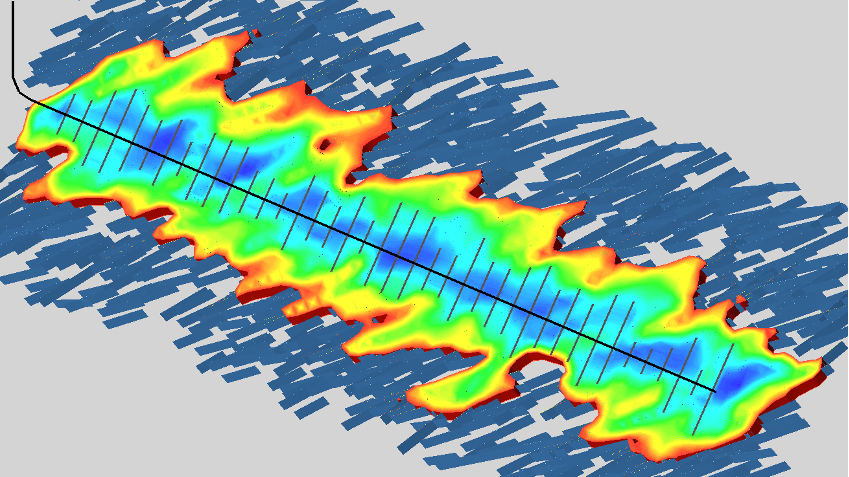
At this point, Datta-Gupta’s research related to the fast marching method (FMM) for fluid front tracking will be added to speed up the system's visual calculations. FMM can rapidly sift through, track and compress massive amounts of data in order to transform the 3D aspect of reservoir fluid movements into a one-dimensional form. This reduction in complexity allows for the simpler, and faster, imaging.
Using known results from recovery processes in actual reservoirs, the researchers will train the system to understand changes the data inputs represent. The system will simulate everyday information, like fluid flow direction and fracture growth and interactions, and show how fast reservoir conditions change during actual production processes.
“We are not the first to use machine-learning in petroleum engineering,” said Datta-Gupta. “But we are pioneering this enhancement, which is not like the usual input-output relationship. We want complex answers, ones we can interpret to get insights and predictions without compromising speed or production time. I find this very exciting.”