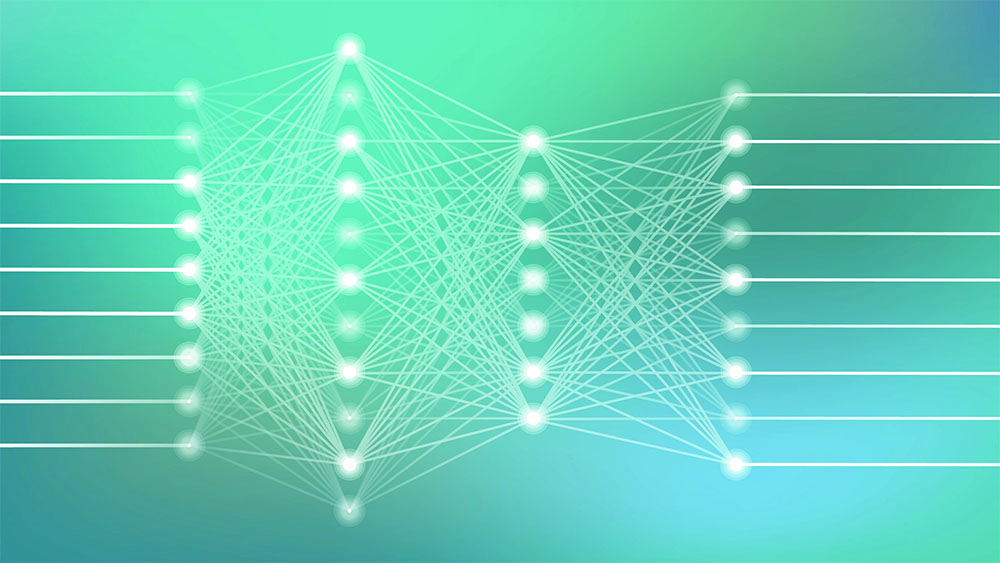
A paper authored by Dr. Joseph Sang-II Kwon, assistant professor; Dr. Arul Jayaraman, professor and head of the Artie McFerrin Department of Chemical Engineering at Texas A&M University; and Dongheon Lee, a doctoral candidate in Kwon’s research group; was selected as an editor's choice paper in the May 2020 AIChE Journal.
In the paper titled “Identification of Cell‐to‐Cell Heterogeneity Through Systems Engineering Approaches,” the researchers offer a new methodology to study a complex problem. Individual cells in genetically homogeneous populations exhibit a significant degree of heterogeneity in their responses to an external stimulus. Essentially, within groupings of the same type of cells, individual cells can exhibit different responses to stimuli. Current approaches to studying this problem have relied on an individual‐based population model (IBPM), where probability density functions (PDFs) are given to model parameters of the IBPM to capture the cell-to-cell heterogeneity. However, there is a lack of a systematic methodology to estimate the PDFs from experiments. Specifically, it is difficult to identify which model parameters are heterogeneous, and once identified it is computationally expensive to estimate their PDFs. Moreover, because of the experimental limitations and nonlinearity of models, not all parameters' PDFs are identifiable.
To deal with the limitations of the current IBPM, the authors utilized process systems engineering concepts to develop a new methodology. Rather than identifying and estimating PDFs of every parameter, only those parameters whose PDFs are identifiable were estimated. Once these parameters were determined, an artificial neural network model was developed to find an empirical relation between these parameters and output PDFs to reduce computational costs of the parameter identification process.