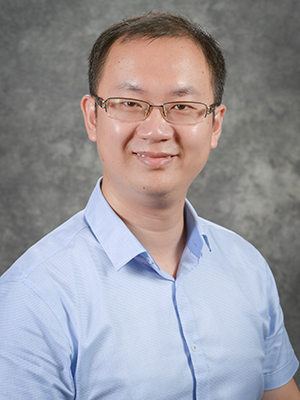
Dr. Xia “Ben” Hu, assistant professor in the Department of Computer Science and Engineering at Texas A&M University, has been selected as a recipient of the J.P. Morgan Artificial Intelligence Faculty Research Award. Through this recognition and grant, Hu will be investigating how to enhance the widespread understanding, impact and credibility of machine learning in industry by creating a system to explain the reasoning behind the decision-making process of a machine.
A leader in finance and one of the world’s most prominent technology banks, J.P. Morgan aims to advance the practical applications of artificial intelligence (AI) by supporting the cutting-edge innovations and investigations of researchers around the globe.
Utilizing algorithms and statistical models, machine learning enables a computer to perform specific tasks without the need for much human interaction. Relying on pattern recognition and inference, this subset of AI can offer critical assistance in real-world problems with applications ranging from helping doctors detect cancer to directing autonomous vehicles to analyzing finance.
While carefully programmed, the lack of a human touch and transparency in AI systems often leads to skepticism and the questioning of ethics regarding machines and their conclusions.
The underlying question becomes why. Why did a machine make a specific diagnosis or predict that the stocks would rise? Why should the results be trusted?
“Although machine learning, especially deep learning, has achieved great success, it is criticized for its black box property — meaning that it is successful, but people don't know why it’s successful and why it achieved such a good performance. Similarly, when it fails, people cannot understand why it fails and that makes it hard to improve,” said Hu.
This missing interpretability, or the extent to which a researcher is able to predict what is going to happen given a change in input or parameters, severely limits the system’s explainability, or the extent to which the internal mechanics of a machine system can be explained in human terms.
Both interpretability and explainability are critical in the advancement and acceptance of machine learning in real-world applications.
With the help of four of his Ph.D. students, Hu is working to improve the widespread understanding of AI by taking a deeper look at machine learning models, algorithms and various data perspectives and developing a way to translate them for the masses. In doing so, his team aims to gain vital insight into the reasoning behind the machine’s decision-making process and conclusions. In turn, this could help solve the mystery as to why machines “think” as they do — giving both researchers and professionals a better understanding of the inner workings of the machine-brain in applications such as anomaly detection and network analytics.
While stepping into the world of finance to enhance machine learning may sound out of place at first, Hu explained that the significance of this partnership is twofold.
First, with all the rich data patterns found in financial systems, it is imperative for industry experts to have a means to quickly and accurately detect things such as fraud or financial anomalies — something that machine learning can easily provide. However, it is equally as important for them to understand why a transaction is labeled as fraudulent or what made the stock predictions change. Hu’s research will bridge the knowledge gap between AI engineers and finance industry experts, leveling the field and allowing everyone to interpret and understand the reasoning behind the system.
Second, through the development of interpretable machine learning algorithms and systems with J.P. Morgan, Hu and his team will be able to apply their research to real-world problems and observe the direct effects of it. In addition to giving them a means to see their research in action and track the results for future improvements, this implementation will also help users have a better and more secure financial experience.
“I am excited and honored to receive this research award and be among so many distinguished faculty members from renown universities,” said Hu. “We have been conducting this research for a few years and are so excited to have the opportunity to work with J.P. Morgan to see how machine learning research could directly contribute to their financial systems.”