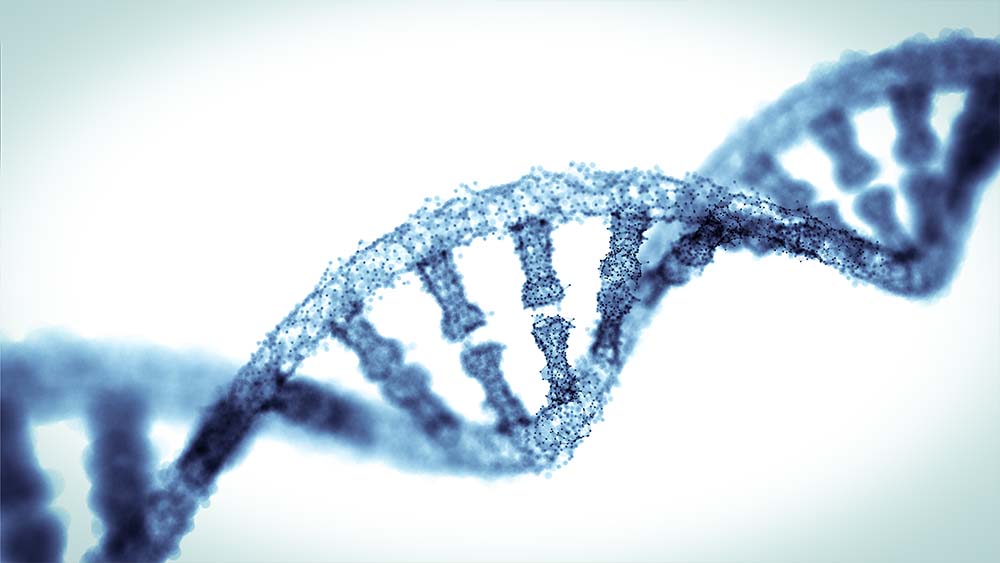
There are certain diseases, such as cancer and Type 1 diabetes, that affect many of us directly. These diseases can not only be difficult to manage, but difficult to reverse, with effects that can be detrimental to a person’s quality of life. Dr. Xiaoning Qian is utilizing signal processing and machine learning tools to decipher which genes are critical to understand and predict disease progression so that biologists can use that information to develop new disease management practices.
Modern biological experiments provide a large amount of data. Big biomedical data involves data sets that are more complex than what the traditional data-processing software can handle. In this case, these diseases offer complex data sets that require appropriate mathematical models and analytic methods to understand.
How genetic differences and environmental stress change the living system is a question Qian is out to answer. In order to begin his analysis, biologists provide him with various affected gene data sets. From there, he and his students develop models and algorithms to analyze the data provided. The goal is to identify important genes and decode which genes are intertwined and which trigger the system response, for example in immune pathways.
“We need to help this gene identification procedure,” said Qian, assistant professor in the Department of Electrical and Computer Engineering at Texas A&M University. “We need to have the statistical methods and computational algorithms to look at the data, to analyze the data, and then try to identify the change specifically due to different genetic and environmental perturbations.”
Qian’s goal is to develop analytic methods leading to biologically meaningful messages that can be validated by other researchers. Ultimately this could lead to the development of a user-friendly software for biologists to easily get the information they need. Right now they are in the early stage of developing methods to effectively analyze genomic data. One way they are working to develop these methods is by incorporating Bayesian methods.
“The idea of Bayesian is that if you base your analysis on a limited number of data samples, there is lots of uncertainty,” Qian said. “You don’t want to ignore that uncertainty – you want to incorporate that uncertainty in your analysis to make sure your derived results are robust.”
Genes are linked in complex ways. This dynamic process can make it difficult for researchers to acquire a full understanding of the story these genes tell. For example, it is important to figure out which genes regulate the immune system to get closer to the desired results. To do this, researchers need to reconstruct the dynamic system in the cells based on their data analysis.
“One potential way to make sure someone can recover is to make sure that even if some critical genes are mutated you can still try to activate the immune system by spiking a similar gene with similar structures,” Qian said. “This is more of a mathematical way to explain the immune system, but in reality, cells may not exactly function that way.”
Qian said that it is important to identify the genes and pathways critical to different complex diseases, to try to understand the relationship among those genes, and then to try to model and simulate to see whether that reflects what you observe in the actual cells.
“Once you identify which genes are actually controllable you can more easily derive genetic therapeutics for the disease,” Qian said.
While this sounds fairly simple in theory, this is a very difficult task in practice. Qian explained that if this can eventually be done, then diseases can be successfully managed or even cured down the road.
The methodology that Qian is developing, which relies on big data set analyses and machine learning algorithms, has application potential well beyond genomics and is well aligned with departmental goals of introducing more data science into the curricula, both undergraduate and graduate.
While Qian is currently working on developing different models and methods for genomic data analysis, the next step is to hopefully have his findings validated across multiple studies. Ultimately, his hope is that the translation of large-scale biomedical data into biological knowledge will help precision medicine to benefit human society.
Modern biological experiments provide a large amount of data. Big biomedical data involves data sets that are more complex than what the traditional data-processing software can handle. In this case, these diseases offer complex data sets that require appropriate mathematical models and analytic methods to understand.
How genetic differences and environmental stress change the living system is a question Qian is out to answer. In order to begin his analysis, biologists provide him with various affected gene data sets. From there, he and his students develop models and algorithms to analyze the data provided. The goal is to identify important genes and decode which genes are intertwined and which trigger the system response, for example in immune pathways.
“We need to help this gene identification procedure,” said Qian, assistant professor in the Department of Electrical and Computer Engineering at Texas A&M University. “We need to have the statistical methods and computational algorithms to look at the data, to analyze the data, and then try to identify the change specifically due to different genetic and environmental perturbations.”
Qian’s goal is to develop analytic methods leading to biologically meaningful messages that can be validated by other researchers. Ultimately this could lead to the development of a user-friendly software for biologists to easily get the information they need. Right now they are in the early stage of developing methods to effectively analyze genomic data. One way they are working to develop these methods is by incorporating Bayesian methods.
“The idea of Bayesian is that if you base your analysis on a limited number of data samples, there is lots of uncertainty,” Qian said. “You don’t want to ignore that uncertainty – you want to incorporate that uncertainty in your analysis to make sure your derived results are robust.”
Genes are linked in complex ways. This dynamic process can make it difficult for researchers to acquire a full understanding of the story these genes tell. For example, it is important to figure out which genes regulate the immune system to get closer to the desired results. To do this, researchers need to reconstruct the dynamic system in the cells based on their data analysis.
“One potential way to make sure someone can recover is to make sure that even if some critical genes are mutated you can still try to activate the immune system by spiking a similar gene with similar structures,” Qian said. “This is more of a mathematical way to explain the immune system, but in reality, cells may not exactly function that way.”
Qian said that it is important to identify the genes and pathways critical to different complex diseases, to try to understand the relationship among those genes, and then to try to model and simulate to see whether that reflects what you observe in the actual cells.
“Once you identify which genes are actually controllable you can more easily derive genetic therapeutics for the disease,” Qian said.
While this sounds fairly simple in theory, this is a very difficult task in practice. Qian explained that if this can eventually be done, then diseases can be successfully managed or even cured down the road.
The methodology that Qian is developing, which relies on big data set analyses and machine learning algorithms, has application potential well beyond genomics and is well aligned with departmental goals of introducing more data science into the curricula, both undergraduate and graduate.
While Qian is currently working on developing different models and methods for genomic data analysis, the next step is to hopefully have his findings validated across multiple studies. Ultimately, his hope is that the translation of large-scale biomedical data into biological knowledge will help precision medicine to benefit human society.