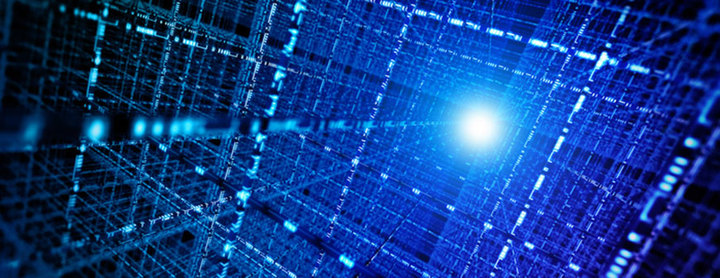
Drs. Yu Ding, Mike and Sugar Barnes professor in the Department of Industrial and Systems Engineering at Texas A&M University; Peng Li, professor in the Department of Electrical and Computer Engineering at Texas A&M University; and Bani Mallick, distinguished professor in the Department of Statistics at Texas A&M University were recently awarded a National Science Foundation (NSF) big data project that aims to develop data science solutions to address the reliability and operational performance issues in wind energy applications.
The NSF big data project is a three-year project. It is called the, "BIGDATA: From Bytes to Watts – A Data Science Solution to Improve Wind Energy Reliability and Operation," and it has a budget of $1.3 million.
The project involves two other partner universities, the University of Michigan and the University of Connecticut. Texas A&M Engineering Experiment Station is the lead institute in this effort. The team also partners with the National Renewable Energy Laboratory (NREL) and a number of renewable energy companies that will provide the crucial access to the operational data of their commercial wind farms.
Despite the remarkable progress that the wind industry has made in the past decade, wind energy is still not market competitive without the substantial federal production tax credit. In order for wind energy to be competitive on its own, its operational performance and reliability needs a significant uplifting through relentless technical innovation. Although a large amount of data is continually being accumulated in the wind industry, making effective use of these heterogeneous wind engineering data is challenging. The primary objective of this NSF big data project is to address this critical need.
“The Energy Assessment Director of a major wind company once told me: 'The potential benefit of data science increases with the amount of available data and the complexity of the system being analyzed. Wind Energy has both: huge amount of data and non-linear and complex characteristics.' I agree with him wholeheartedly,” said Ding. “With the talents in this multidisciplinary team, I am excited about the potential impact of our data science solutions, which could enable wind energy to stand on its own feet."
Working closely with domain experts in the wind industry, Ding's team plans to develop data science solutions that evaluate and improve a wind turbine’s design and operation, enhance the understanding of local wind field dynamics and the short-term wind forecasting, optimize for maintenance policies under weather uncertainty and disruption, as well as integrate these data science solutions into a reliable and efficient computational platform.
Ding is jointly appointed with the Department of Electrical and Computer Engineering. His research specializations include system informatics, data and quality science, and their applications in wind energy and manufacturing.
Li specializes in integrated circuits and systems, machine learning and its hardware realization in very-large-scale integration. He specializes in integrated circuits and systems, brain-inspired computing, machine learning and its hardware realization in very-large-scale integration.
Mallick holds the Susan M. Arseven'75 Chair in Data Science and Computational Statistics. He is an internationally renowned expert in the field of Bayesian predictive modeling and uncertainty quantification.