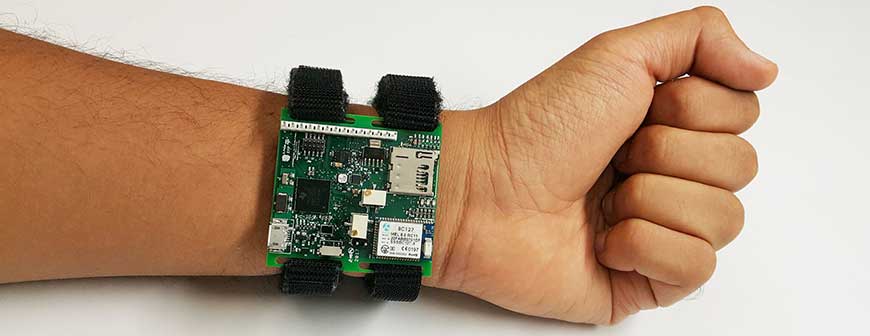
To solve a problem you often need to find a pattern. The deeper understanding researchers have of the factors and implications of clinical conditions, the closer they are to offering prevention and better care practices to patients.
Dr. Bobak Mortazavi, assistant professor in the Department of Computer Science and Engineering at Texas A&M University, is working on a project to develop medical sensors that produce data for tracking risk factors for various cardiovascular diseases, such as heart attacks and heart failure.
Mortazavi is using data mining, which is a way of examining large data sets in order to generate new information, and machine learning applications to design clinical decision support systems and research clinical outcomes.
“We can create models to predict the risk of complications for a patient using data available in clinical records, as well as personal and wearable sensors,” Mortazavi said. “Then we use these outcome models to lead the design of new sensing systems in order to create dynamic, personalized predictions based upon each patient’s daily data.”
By developing medical sensors to track these risk factors on a daily basis, Mortazavi and his team have the ability to better understand long-term modeling of biomedical data, to understand and design metrics for improvement in care, and to potentially identify new characteristics of clinical conditions to improve personalized patient care.
The knowledge of the medical field is not enough to understand the engineering and development of the tools. On the flip side, understanding clinical research also is not solely useful for developing the newest sensing and learning techniques. Without thorough knowledge of each, certain advancements would simply be impossible.
“The importance of this work is in its truly interdisciplinary nature,” Mortazavi said. “Through our collaborations, we aim to train researchers in all components of this end-to-end research. From the sensing systems, we teach how users collect data; to the data mining and machine learning applications, we teach them to identify clinically-relevant patterns in data. Most importantly, we all learn to understand the lessons from this data to assist in clinical decision-making and increase the potential of improving patient care.”
Mortazavi first began studying this topic in 2014 when he completed his postdoctoral studies at the Yale School of Medicine. His doctorate studies involved human activity recognition, wearable sensors and exergaming, the development of gaming applications in user cyber physical systems to encourage activities with sports-levels of intensity. While those systems worked in short spurts, he became interested in learning how to apply such systems for longer-term use for clinical utility.
These studies include learning how long-term use of the systems can affect clinical adverse events, how to identify risks of those events, and how to design the user interfaces to such systems so the patients and clinicians can have improved decision-making about trajectories of care.
Mortazavi, who is a member of the Texas A&M Engineering Experiment Station (TEES) Center for Remote Health Technologies and Systems, is collaborating with researchers and faculty members within the center and the department, such as Dr. Atlas Wang, assistant professor; Dr. Theodora Chaspari, assistant professor; Dr. Ricardo Gutierrez-Osuna, professor; and Dr. Roozbeh Jafari, associate professor in computer science, biomedical, and electrical and computer engineering.
He also has collaborators outside of Texas A&M, such as Dr. Harlan Krumholz, who serves as the Harold H. Hines, Jr. Professor of Medicine (Cardiology) and professor in the Institute for Social and Policy Studies, of Investigative Medicine and of Public Health (Health Policy); director of the Center for Outcomes Research and Evaluation at Yale-New Haven Hospital; and co-director of the Robert Wood Johnson Foundation Clinical Scholars Program at Yale University.
Though he and his team are currently focused on cardiovascular diseases, Mortazavi hopes to branch out to a number of other health conditions in the future.