From the healthcare industry, to wind energy, the oil and gas industry and manufacturing, researchers from the system informatics group in the Department of Industrial and Systems Engineering at Texas A&M University are developing data-driven methods to improve the systems and processes involved with each area.
“We take in data and solve engineering systems by developing sophisticated data analytics methods while considering engineering domain knowledge and models,” said Dr. Yu Ding, professor and senior member of the group. “System informatics fuses system knowledge into data modeling and analysis. It is a key area in addressing the challenges of big data.”
Serving Efficiently
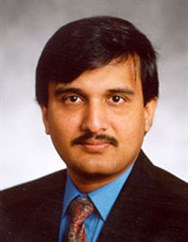
As a result of the Affordable Care Act, the call for improved access to primary care for all Americans has reached an all-time high. Additionally, there has been a nationwide trend amongst healthcare providers to transform their practices to become Patient Centered Medical Homes (PCMH), with the primary emphasis on improving quality of care. A key function of PCMH is accessible service that requires improving accessibility of primary care for patients.
With 90 percent of patient care delivered through out patient services, appointment schedules must be improved to ensure the success of all patients. Dr. Amarnath Banerjee, associate professor, is working with his Ph.D. student, Yu Fu, to develop models that will enable a clinic to serve the right mix of traditional patients and same day request patients, which will improve timely access to primary care.
“The objective is to develop a balanced schedule that tends to minimize both the waiting time for patients as well as idle time for physicians,” Banerjee said. “The waiting time increases if there are too many patients that are scheduled while the idle time increases if there are not enough patients.”
This problem becomes difficult to solve in a one size fits all manner. Banerjee and his team use stochastic factors, estimated using advanced statistical techniques, to account for each clinic’s individual characteristics. These factors include patients arriving late or early for their appointments, patients not showing up for their appointments, the variability associated with the encounter time between a patient and the physician, and not completely knowing how many same day requests to expect on a given day.
“The model uses existing clinic-specific information available for the previously scheduled traditional patients and the stochastic factors to develop a daily schedule for the clinic,” Banerjee said. “In the process, the model also determines the value of the available information and the extent it helps in developing the appropriate schedule for the clinic.”
This research will help practice managers develop better appointment schedules for their clinic using the clinic’s own data, and will improve accessibility and quality of care for its patients.
Understanding wind speed
According to a report from the Renewable Energy Policy Network for the 21st Century, countries worldwide invested $286 billion in renewable energy and about 7.7 million jobs around the world were associated with the industry in 2015.
Wind energy is a type of variable renewable energy, which means the primary energy source, the wind, and other influencing environmental factors — air density, humidity and atmospheric stability — cannot be controlled by people. Therefore, the wind power output of a turbine is constantly changing.
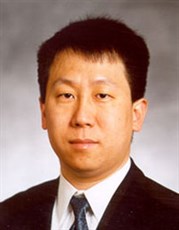
As a result of this variable nature, the Big Data-driven predictive analytics is needed by wind energy producers and wind farm operators for decision making. These analytics allow the producers and operators to understand how the wind field is changing or if the turbines are performing to the design standards. Dr. Yu Ding and his research group are improving the reliability of wind power production to enhance the market’s competitiveness.
“Our group has been working closely with practitioners in the wind industry and received over 100 gigabytes of wind farm operational data,” Ding said. “The large amount of data provides an unprecedented opportunity for us to address some critical technical challenges in wind energy predictive analytics.”
Ding’s team is developing statistical and machine learning models to understand the local wind field and its dynamics to help farm operators forecast wind speed more accurately in the future.
“We also use the in-field measurements of both power outputs and environmental inputs, wind speed included, to understand how a turbine performs,” Ding said. “Answering these predictive analytics questions helps the operators plan maintenance actions to counter turbine degradation, justify costly retrofitting turbine upgrades, or optimize turbine control for better production capability or a longer service life.”
The group has received grants from the Texas A&M Energy Institute and the National Science Foundation to perform research in this field.
Developing Success
Almost 20 million barrels of oil are used each day in the United States. Therefore, extracting oil from the earth is a process that must occur in a timely manner.
Dr. Andrew Johnson, associate professor, is working to develop a manufacturing system for National Oilwell Varco (NOV). This system will allow it to be the world’s leader in the manufacturing of complex, highly engineered oil extraction equipment. This project is in collaboration with researchers from the University of Wisconsin-Madison and Pennsylvania State University.
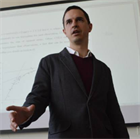
Constructing land or subsea drilling systems is a very lengthy and expensive process. Additionally, the industry is ever changing. A year and a half ago, the demand for drilling was very high, but today, there is very little demand.
“NOV’s manufacturing processes are making significant improvements,” Johnson said. “The goal of this project is to develop an integrated manufacturing system for NOV to build complex highly customized products that will allow them to be as successful as Toyota was with the mass production of cars.”
To accomplish this goal, the researchers have identified three critical areas for development.
“These areas are manufacturing organization, measuring manufacturing performance and manufacturing processes,” Johnson said. “Texas A&M is leading the research in measuring manufacturing performance.”
The Texas A&M team is comprised of Johnson and four graduate students. Over the past five years, 11 students have worked with six different plants and saved the company millions of dollars through improved communications and efficiency in operations.
“We have focused on improving the integration of shop floor metrics with the overall objectives of the plants and company,” Johnson said.
While the oil industry is currently in a downturn, this is the right time to apply improved measurement and operational practices to continue to allow NOV to be the world’s leading provider of equipment and components used in oil and gas industry.
Building Quality Control
Dr. Li Zeng, assistant professor, analyzes and monitors manufacturing and healthcare delivery systems for improvement and quality control.
“The concerns on energy and environment during the past two decades have led to improvements in house and building design,” Zeng said. “This is made possible through the use of energy efficient windows made of low-emittance glass.”
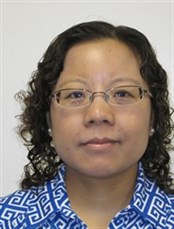
Low-emittance glass can reduce unwanted heat gain in the summer and heat loss in the winter by reflecting up to 90 percent of infrared radiation.
“One study suggests that if all windows are made of such glass, the collective annual energy bill of our nation could be reduced by 25 percent per year,” Zeng said. “This has made low-emittance glass manufacturing an important sector of the glass industry.”
The current quality control methods are unreliable because of the simple use of quality measurements such as the measurement of optical properties at different locations of the glass surface. Essentially, the low emittance glass industry is a data-rich environment due to the application of advanced information and sensing technologies in recent years. Unfortunately, the traditional quality control system is not able to integrate the data collected with the process monitoring and root cause diagnosis efforts.
“I am developing a method that will make full use of the information in the measurements, thus being able to provide more reliable results,” Zeng said. “For example, when quality problems occur, current methods may still report false information that the manufacturing process is running normally. My method will generate a signal of process change.”
Zeng’s work on process control in glass manufacturing was awarded the Best Applications Paper award by the Institute of Industrial and Systems Engineers. The paper, titled “Bayesian Hierarchical Modeling for Monitoring Optical Profiles in Low-E Glass Manufacturing Processes,” was featured in the Industrial Engineering (IE) Magazine.
Changing the world
Drs. Banerjee, Ding, Johnson and Zeng are conquering processes in their respective industries to improve the life we know today.
As a result of their work, patients will get care when they need it and avoid costly emergency room visits and hospital admissions. Wind energy will become more available, resulting in cheaper energy rates with decreased effects on the environment. The oil and gas industry will be capable of producing highly customized equipment at a lower cost and in a timely manner, making them more accessible to consumers quicker. By creating a higher quality low emittance glass, the home or business owner will benefit from big savings in energy use.
Whether directly or indirectly, every project will impact our daily lives for the better.