- Assistant Professor, Industrial & Systems Engineering
- 2022 Texas A&M Institute of Data Science Career Initiation Fellow
- Affiliated Faculty, Department of Statistics
- Phone: 979-458-2345
- Email: ruituo@tamu.edu
- Office: ETB 4012
- Website: Personal Website
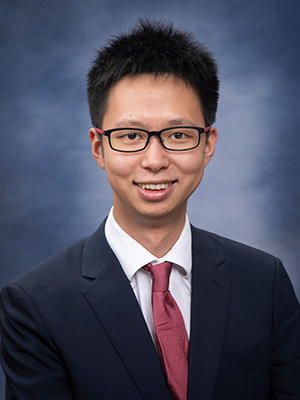
Educational Background
- Ph.D., Statistics, University of Chinese Academy of Sciences, 2013
Research Interests
-
- Design and analysis of computer experiments
- Uncertainty quantification
- Statistical inference in random fields
- Nonparametric and semiparametric statistics
- Bayesian inference and computation
Awards & Honors
- Career Initiation Fellow, Texas A&M Institute of Data Science, 2022
Selected Publications
- Tuo, R. and Wang, W., (2022) "Uncertainty Quantification for Bayesian Optimization," The 25th International Conference on Artificial Intelligence and Statistics, accepted.
- Prakash, A., Tuo, R., and Ding, Y., (2021+) “Gaussian Process Aided Function Comparison Using Noisy Scattered Data,” Technometrics, to appear.
- Tuo, R., He, S., Pourhabib, A., Ding, Y., and Huang, J., (2021+) “A Reproducing Kernel Hilbert Space Approach to Functional Calibration of Computer Models,” Journal of the American Statistical Association, to appear.
- Zhang, W., Krehbiel, S., Tuo, R., Mei, Y., and Cumming, R., (2021) “Single and Multiple Change-Point Detection with Differential Privacy,” Journal of Machine Learning Research, 22(29):1−36.
- Wang, Y., Yue, X., Tuo, R., Hunt, J. H., and Shi, J., (2020) “Effective Model Calibration via Sensible Variable Identification and Adjustment, with Application to Composite Fuselage Simulation,” Annals of Applied Statistics, 14(4), 1759-1776.
- Tuo, R., and Wang, W., (2020) “Kriging prediction with isotropic Matérn correlations: robustness and experimental design,” Journal of Machine Learning Research, (187), 1−38.
- Wang, W., Tuo, R., Wu, C. F. J., (2020) “On Prediction Properties of Kriging: Uniform Error Bounds and Robustness,” Journal of the American Statistical Association, 115(530), 920-930.
- Ding, L., Tuo, R., Shahrampour, S., (2020) “Generalization Guarantees for Sparse Kernel Approximation with Entropic Optimal Features,” In International Conference on Machine Learning (ICML), (pp. 2875-2884).
- Tuo, R., (2019) “Adjustments to Computer Models via Projected Kernel Calibration,” SIAM/ASA Journal on Uncertainty Quantification, 7(2), 553-578.
- Tuo, R., and Wu, C. F. J., (2018) “Prediction Based on the Kennedy-O’Hagan Calibration Model: Asymptotic Consistency and Other Properties”, Statistica Sinica, 28(2), 743-759
- Tuo, R., and Wu, C. F. J., (2016) “A Theoretical Framework for Calibration in Computer Models: Parametrization, Estimation and Convergence Properties,” SIAM/ASA Journal on Uncertainty Quantification, 4(1), 739-766
- Tuo, R., and Wu, C. F. J., (2015) “Efficient Calibration for Imperfect Computer Models,” The Annals of Statistics, 43(6), 2331-2352
- Plumlee, M., and Tuo, R. (2014) “Building Accurate Emulators for Stochastic Simulations via Quantile Kriging,” Technometrics, 56(4), 466-473
- Tuo, R., Wu, C. F. J., and Yu, D. (2014) “Surrogate Modeling of Computer Experiments with Different Mesh Densities,” Technometrics, 56(3), 372-380